Keynote
David Lazer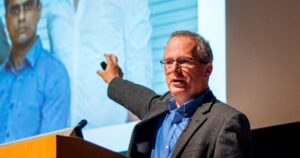
Building a trans-Atlantic coalition for studying the giants of the internet
This presentation will critically discuss the research paradigms that have dominated the study of the internet over the last decade, how those paradigms are currently in collapse, and how we need to build a trans-Atlantic effort to study the giants of the internet that increasingly dominate informational economies of the US and Europe.
David Lazer is University Distinguished Professor of Political Science and Computer Sciences, Northeastern University, faculty fellow at the Institute for Quantitative Social Science at Harvard, and elected fellow of the National Academy of Public Administration. He has published prominent work on computational social science, misinformation, democratic deliberation, collective intelligence, and algorithmic auditing, across a wide range of prominent journals such as Science, Nature, Proceeding
Session 1: Complexity Science for Social Science
David Garcia
Language Understanding as a Constraint on Consensus Size in LLM Societies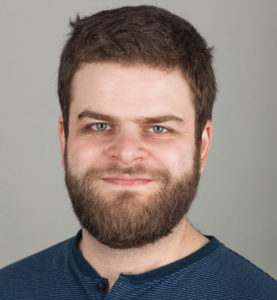
The applications of Large Language Models (LLMs) are going towards collaborative tasks where several agents interact with each other like in an LLM society. In such a setting, large groups of LLMs could reach consensus about arbitrary norms for which there is no information supporting one option over another, regulating their own behavior in a self-organized way. In human societies, the ability to reach consensus without institutions has a limit in the cognitive capacities of humans. To understand if a similar phenomenon characterizes also LLMs, we apply methods from complexity science and principles from behavioral sciences in a new approach of AI anthropology. We find that LLMs are able to reach consensus in groups and that the opinion dynamics of LLMs can be understood with a function parametrized by a majority force coefficient that determines whether consensus is possible. This majority force is stronger for models with higher language understanding capabilities and decreases for larger groups, leading to a critical group size beyond which, for a given LLM, consensus is unfeasible. This critical group size grows exponentially with the language understanding capabilities of models and for the most advanced models, it can reach an order of magnitude beyond the typical size of informal human groups.
David Garcia studied Computer Science at Universidad Autonoma de Madrid and ETH Zurich, where he also did his Doctorate and Habilitation at the Department of Management, Technology, and Economics. He has contributed to the development of interdisciplinary master’s programs and teaches master-level courses in Social Data Science, Computational Modelling of Social Systems, and Large Language Models. His research focuses on the analysis of digital traces to study human behavior and to model human societies with a combination of complexity science methods and generative language models.
Chico Camargo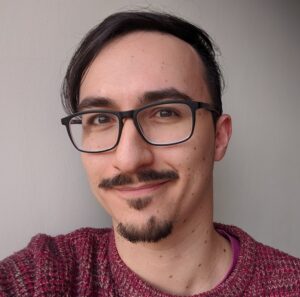
Complexity Science for Social Science: Unpacking the Dynamics of Social Systems
Complexity science, which focuses on understanding the behaviour of interconnected and adaptive systems, provides powerful tools for exploring the multifaceted nature of social phenomena. In this presentation, we will discuss how principles of complexity — such as emergence, network dynamics, and self-organization — can offer unique insights into social science challenges. We will examine case studies where complexity science has successfully explained patterns in social behaviour, from epidemic modelling to economic networks, demonstrating how these concepts can be adapted to tackle contemporary social issues. We will highlight open research questions and opportunities for interdisciplinary complexity scientists to work in the social sciences and collaborate with social scientists. Join us to discover how complexity science can be an invaluable ally in deepening our understanding of social systems and promoting a more informed and equitable society.
Chico Camargo is a Senior Lecturer in Computer Science at the University of Exeter. He is also the Computational Social Science Theme Lead at the Institute of Data Science and Artificial Intelligence, Deputy Director at the Centre for Climate Communication and Data Science, Research Associate at the Oxford Internet Institute, University of Oxford, Visiting Professor at the Department of English Language and Literature, Ewha Womans University, Seoul, Turing Fellow at the Alan Turing Institute, and director of the CC Lab. He studies how ideas spread and evolve, mixing data science with theories about human behaviour, culture, and society. He is also a science communicator, having already written for Science, HuffPost Brasil, The Conversation, and produced more than 50 videos for YouTube.
Margarita Torre
Chasing the Unicorn: Reflections on the Training of Computational Social Scientists
This talk discusses the challenges of training the next generation of computational social scientists, balancing the complexities of delimiting the discipline with the promising opportunities for growth and innovation in this rapidly evolving field.
Margarita Torre is a Professor of Sociology and Co-Director of the master’s program in Computational Social Sciences at Universidad Carlos III de Madrid. Her research focuses on labor inequalities and quantitative data analysis. She has published in leading international journals such as Social Forces, PLOS ONE, Work and Occupations, and Gender and Society. Currently, she serves as an Associate Editor for European Societies, the official journal of the European Sociological Association. Margarita is also actively engaged in public outreach, contributing to blogs like Work in Progress and the LSE Business Review, as well as Spanish platforms such as the Observatorio Social de “la Caixa”, Nada es Gratis, and Piedras de Papel.
Session 2: Data Science and AI for Social Science
Hannes Mueller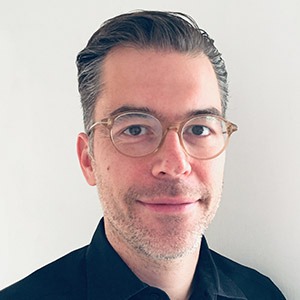
Prediction Policy Problems Require us to Integrate Ethics, Machine Learning and Causal Inference
Prediction policy problems such as the collapse of political institutions, hate speech, crime, pandemics, economic crisis or armed conflict, are key problems for society. This intervention will offer a summary of research on the prediction and prevention of armed conflict. To make further progress in this area, we need to solve a dual problem of simultaneous forecasting and treatment effect estimation under ethical constraints. The problem is well-known in ethics and the social sciences but needs to be mapped out in a new way as advances in machine learning and increased data availability have led to an explosion in our capacity to forecast without causal models.
Hannes Mueller is a tenured researcher at the Institute for Economic Analysis (IAE-CSIC) and the Director of the Data Science for Decision Making Program at the Barcelona School of Economics (BSE). He is affiliated with CEPR and serves as the theme leader for Institutions, Democracy, and Peace in the RECIPE project. His research focuses on the application of machine learning techniques to issues in Political Economy, Conflict Studies, and Development Economics. Hannes’ work often involves analyzing large datasets, such as newspaper archives and satellite images, to forecast and nowcast violence, with publications in prominent journals like the American Economic Review (AER), American Political Science Review (APSR), Proceedings of the National Academy of Sciences (PNAS), and the Journal of the European Economic Association (JEEA).
Hannes has contributed extensively to public policy through research projects commissioned by various governments and international organizations, including the World Bank, IMF, UN, several INGOs, the Banco de España, the Foreign, Commonwealth & Development Office, and the German Federal Foreign Office. His work spans topics such as political transitions, displacement, the economic effects of armed conflict, structural transformation, and studies on political risk, conflict forecasting, and conflict prevention. Through projects like conflictforecast.org and the EconAI research group, Hannes combines academic research at the intersection of text mining, machine learning, and causal inference with public outreach. His research has featured in newspapers like the Financial Times, El Pais, La Vanguardia, national radio, and several podcasts, and blogs.
Shaily Gandhi
Enhancing Disaster Response with Social Media Analytics
The convergence of data science and social science offers unprecedented opportunities to address pressing societal challenges, with disaster management being a prime example. The TEMA project, funded by the European Union, seeks to revolutionise natural disaster management by harnessing the power of artificial intelligence for real-time analysis of extreme data, including geosocial media content.
This presentation will focus on the social science implications of the TEMA project, specifically exploring the role of AI in analysing geosocial media during disaster events. It will showcase the development and implementation of novel semantic analysis algorithms.
Shaily is a Postdoc at Geo-Social Analytics Lab, Department of Geoinformatics – Z_GIS, University of Salzburg. She is a GIS expert with more than 12 years of experience. She has a PhD from CEPT University, India in Geospatial Technology and has expertise in bridging the gap between GIS & governance. Shaily has been awarded the Geospatial World 50 Rising Star for the year 2023 by Geospatial World and ISC Fellow for 2024. Shaily has led CODATA Connect and also has been CODATA Excom member. Shaily is keen on exploring the implementation of GIS and data science in the domain of Urban Analytics. Her core interest lies in exploring spatial technology for better decision-making along with defining spatial data standards for data interoperability for building future cities.
Sebastian Poledna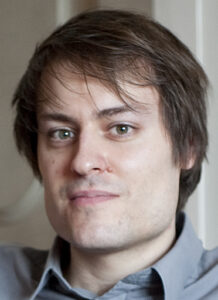
Economic Modelling with High-Performance Computing
The presentation showcases the IIASA macroeconomic agent-based model, which integrates micro and macroeconomic data, providing forecasts competitive with standard models, such as VAR and DSGE. Applications, including the 2007-2008 financial crises, the COVID-19 pandemic, the post-pandemic inflation surge, and labor market dynamics in response to migration, demonstrate the practical application of the ABM in forecasting and understanding economic trends. Finally, the presentation discusses technical challenges and solutions for implementing large-scale simulations using high-performance computing.
Sebastian Poledna is the leader of the Exploratory Modeling of Human-natural Systems (EM) Research Group of the IIASA Advancing Systems Analysis Program. His scientific interests include new approaches to macroeconomics, the impact of climate change on socioeconomic systems, the systemic risk of various complex systems, and financial regulation. He holds double degrees in physics (2005-2011) and in economics and business administration (1999-2003), and has worked as a practitioner in risk management at one of the largest European banks for almost a decade (2007-2015). He obtained his PhD in physics at the University of Vienna in 2016. Poledna first joined IIASA as a research scholar in the former IIASA Advanced Systems Analysis and Risk and Resilience programs in 2015. In January 2021, he was appointed research group leader of the EM Research Group. The EM Research Group currently has 35+ scientists whose aim is to produce methodological advances that will underpin future IIASA research.
Session 3: Policies and technical advances to maximize access
Stefano Iacus
Enhancing FAIRness in Harvard Dataverse with Variable-Level Metadata and Differential Privacy
We highlight recent advances in the Dataverse Project aimed at extending support for variable-level metadata beyond tabular data, incorporating DDI, CDI-DDI, CroissantML, and other schemas to enhance discoverability and interoperability. Additionally, we explore the integration of differential privacy techniques to improve access to sensitive data while maintaining confidentiality, thereby promoting broader accessibility and compliance with FAIR principles.
Stefano M. Iacus is the Director of Data Science and Product Research at the Institute for Quantitative Social Science (IQSS), Harvard University. He also serves as the Managing Director of the Dataverse Project and is a member of the executive committee of the OpenDP Project. In addition, he oversees the Data Science Services and the Data Acquisition & Archiving teams at IQSS. Iacus is an affiliate faculty member of the Kempner Institute for the Study of Natural and Artificial Intelligence at Harvard.
Iacus began his academic career at the University of Milan (Italy), where he became a full professor of statistics in 2015. He founded and directed the Data Science Lab and created two master’s programs in Finance and Economics, and Data Science for Economics. From 2019 to 2022, he served as an officer at the Joint Research Centre of the European Commission, leading a team that leveraged non-traditional data sources for evidence-based policy-making in areas like migration and demography, particularly during crises and to improve preparedness measures.
Since 2006, Iacus has held a recurring visiting position at the Graduate School of Mathematics at the University of Tokyo, where he co-leads the Yuima Project. He was a member of the R Core Team from 1999 to 2014 and remains a member of the R Foundation for Statistical Computing.
Beyond academia, Iacus played a critical role during the COVID-19 pandemic, managing a large-scale business-to-government project for the European Commission. This initiative used data from mobile network operators to generate insights for policy-making across European Union member states.
Iacus has published numerous books, scientific articles, and open-source software products, covering fields such as causal inference, sentiment analysis, stochastic processes, computational statistics, and quantitative finance. His work is widely cited, and he has founded two startup companies in social media analysis and quantitative finance.
Darren Bell
Putting the A back in FAIR: Approaches to finally solving the Access problem.
For too long, access to data has either been the province of cybersecurity professionals or written off as administrative & legal issue that’s inimical to machine-actionability and automation. This presentation outlines how we need to re-think what access actually means in terms of risk appetite, how repositories’ handling of digital objects needs an overhaul and why we must embrace metadata and machine learning at scale in order to enable researchers to focus on doing research.
Darren Bell has worked at the UK Data Service for twelve years, firstly as a data modeler/developer and as Director of Technical Services since the beginning of 2020. Prior to 2012, he worked in a variety of roles in both global infrastructure and development in both the public and commercial sectors. His special interests are linked open data, metadata standardization – especially vocabularies and ontologies – and the automation of traditionally administrative practices like access and rights management. He is vice-chair of the DDI Scientific Board and has championed the implementation of DDI-CDI at the UK Data Archive as a key technology in enabling real-world data interoperability for the future.
Session 4: Data description for AI and machine analysis
Gyorgy Gyomai
SDMX as an enabler for AI applications
SDMX, short for Statistical Data and Metadata eXchange is a standard designed in the early 2000s to facilitate data and metadata exchange between National Statistics offices and international organisations. Since then, the design scope has broadened to include the representation of the data at various stages of the data production cycle, and to a certain degree the cycle itself. The goals of the standard – similar to Linked Open Data – is to improve connectivity, machine readability and facilitate harmonisation and data integration. While SDMX delivers on its goals, in domains such as data discoverability, and connectivity beyond the SDMX world, it can be certainly improved. We will look into exploratory work where AI has been used to augment the discoverability, and recall of the data in SDMX powered data-warehouses.
Gyorgy (Gyomai) is leading the Smart Data Practices team in the Statistics directorate of OECD. He is an economist, statistician, and most recently a data modeller. Over the last years he and his team has assisted data producers within the OECD and the broader community of national statisticians to re-design and harmonise their structural metadata and improve their data production workflows.
Elena Simperl
Croissant: A metadata format for AI-ready datasets
Data is a critical resource for machine learning (ML) in any application domain, yet working with data remains a key friction point. I will introduce Croissant, a metadata format for datasets that creates a shared machine-readable representation across ML tools, frameworks, and platforms. Croissant is open-source and stewarded by a community of volunteers in MLCommons. Informed by existing dataset documentation approaches like data cards, it makes datasets more discoverable, portable, and interoperable, thereby addressing ongoing challenges in ML data engineering. Croissant is already supported by several popular dataset repositories, spanning hundreds of thousands of datasets, enabling easy loading into the most commonly-used ML frameworks, regardless of where the data is stored. Our initial evaluation shows that Croissant metadata is deemed readable, understandable, complete, yet concise by human raters. Croissant is extensible by design, with some extensions under development to address use cases in responsible AI, health, and geospatial information systems.
Elena Simperl is a Professor of Computer at King’s College London and the Director of Research for the Open Data Institute (ODI). She is a Fellow of the British Computer Society and the Royal Society of Arts, and a Hans Fischer Senior Fellow. Elena’s work is at the intersection between AI and social computing. She features in the top 100 most influential scholars in knowledge engineering of the last decade and in the Women in AI 2000 ranking. She is the president of the Semantic Web Sciences Association.
Christine Kirkpatrick
Dimensions of AI Readiness – New Methods and Architectures
With the field of AI rapidly evolving, the notion of what it means for data to be AI ready is continuously shifting. Even so, key steps are clear, some dating back to datamining and some unique in the context of deep learning’s LLMs and Gen-AI technologies. This talk will be informed by the latest community thinking from the FARR community: FAIR in Machine Learning (ML), AI Readiness, AI Reproducibility, a Research Coordination Network funded by the US National Science Foundation NSF). FAIR Digital Objects (FDOs) and their ability to bring together metadata and data stored in different locations by different owners will also be discussed for its potential utility in the social sciences.
Christine Kirkpatrick leads the San Diego Supercomputer Center’s (SDSC) Research Data Services division, which manages large-scale infrastructure, networking, and services for research projects of regional and national scope. Her research is in data-centric AI, working at the intersection of ML and FAIR, with a focus on making AI more efficient to save on power consumption and ‘time to science’. Kirkpatrick serves as PI of the FAIR in ML, AI Readiness & Reproducibility RCN which focuses on promoting better practices for AI, improving reproducibility, and exploring research gaps in data-centric AI. In addition, Kirkpatrick founded the GO FAIR US Office, is PI of the West Big Data Innovation Hub, is on the Executive Committee for the Open Storage Network, and is Co-PI of the NSF-funded Transboundary Groundwater Resiliency (TGR) network. Christine serves on the National Academies of Sciences, Engineering, and Medicine’s Board on Research Data and Information (BRDI) to improve the stewardship, policy and use of digital data and information for science and the broader society. She serves as the Secretary General of the International Science Council’s Committee on Data (CODATA), co-Chairs the FAIR Digital Object Forum, is on the Advisory Board for the Helmholtz Federated IT Services (HIFIS), and serves on the National Academies of Sciences’ U.S. National Committee for the Committee on Data.
Session 5: Provenance, lineage and reproducibility
Tony Ross-Hellauer
Reproducibility challenges in Computational Social Science: Insights from the TIER2 Project
Concerns about the robustness and credibility of research findings—often termed a “reproducibility crisis”—have emerged across many disciplines in recent decades. Broadly, reproducibility refers to achieving consistent results when repeating experiments or analyses. It is usually taken as a key tenet of science itself, if not a direct proxy for quality of results. Addressing this issue requires both technical improvements like consistent and transparent methods and documentation, as well as tackling social factors like questionable research practices and publication bias. While many challenges are shared across fields, the diversity of research methods and cultures (“epistemic diversity”) necessitates tailored approaches. In this talk, I will outline reproducibility challenges specific to computational social science (CSS) and introduce first results from the TIER2 project’s work to develop a researcher checklist, designed to enhance reproducibility in CSS.
Tony Ross-Hellauer is Head of the Open and Reproducible Research Group, an interdisciplinary research group based at Graz University of Technology and Know Center Research GmbH, located in Graz, Austria. A meta-researcher with a background in Information Science and Philosophy, his research focuses on a variety of topics related to the evaluation and governance of research, with a specific focus on Open Science. He is Deputy Chair of the UK Reproducibility Network International Advisory Committee and Project Coordinator and PI for the EC-Horizon project TIER2.
Carole Goble
FAIR Digital Objects for Reproducible Computational Processing
Computational multi-step processing goes by many names – workflows, scripts, pipelines, toolchains, notebook flows etc. By recording the components needed for execution we can make reproducible (transparent) methods, and we can track the provenance of the resulting data products. How do we make that record? How do we package all the components into the FAIR Digital Objects needed for archiving, sharing and comparing a computational process? Is there a framework that standardizes the key components needed to enable reproducibility across computational science disciplines? Yes, there is. In this talk I will introduce the RO-Crate FAIR Digital Object framework for Reproducible Computational Processing.
Carole Goble is a Full Professor of Computer Science at the University of Manchester where she leads the eScience Group of Researchers, Research Software Engineers and Data Stewards. She has 30+ years’ experience of leading digital research infrastructure development, and advancing innovations in research reproducible science, open and FAIR data and method sharing, knowledge and metadata management and computational workflows in a range of disciplines, notably the Life, Biodiversity and Health Sciences. Carole is the Joint Head of Node of ELIXIR-UK the national node of ELIXIR Europe, the European Research infrastructure for Life Science data. ELIXIR has 25 national nodes and 240+ organisations. She is responsible for ELIXIR flagship services for workflow sharing, and FAIR digital object metadata middleware. Carole is also co-Scientific Director of Federated Analytics for Health Data Research UK, a founder of the UK’s Software Sustainability Institute, a partner in numerous projects in the European Open Science Cloud, and currently serves as the UK expert representative on the G7 Open Science Working Group.
Rosa Badia
Workflow programming support for Computational Sciences
Computational Social Sciences is one of the fields among others which can express their applications through workflows. At the Barcelona Supercomputing Center we have been working on PyCOMPSs a programming environment which supports the development of workflow applications which can then be parallelized at execution time and run in distributed computing platforms. Also, PyCOMPSs has been extended to help on the development of applications that combine High-Performance Computing, Artificial Intelligence and Big Data. The talk will overview PyCOMPSs, its main features with an emphasis on how workflow provenance can be automatically recorded with RO-CRATEs and how workflows can be re-executed thanks to this object; the talk will also describe dislib, a machine learning library parallelized with PyCOMPss. We will illustrate the talk with a demographic study application from our Social Sciences Unit and our plans to support the Social Sciences Community further.
Rosa M. Badia holds a PhD in Computer Science (1994) from the Technical University of Catalonia (UPC). She is the manager of the Workflows and Distributed Computing research group at the Barcelona Supercomputing Center (BSC, Spain). Her research has contributed to parallel programming models for multicore and distributed computing. Recent contributions have been focused in the are of the digital continuum, proposing new programming environemnts and software environment for edge-to-cloud. The research is integrated in PyCOMPSs/COMPSs, a parallel task-based programming distributed computing framework, and its application to developing large heterogeneous workflows that combine HPC, Big Data, and Machine Learning. The group is also doing research around the dislib, a parallel machine learning library parallelized with PyCOMPSs. Dr Badia has published nearly 200 papers on her research topics in international conferences and journals. She has been very active in projects funded by the European Commission and in contracts with industry. She has been the PI of the EuroHPC project eFlows4HPC.
She is a member of HiPEAC Network of Excellence. She received the Euro-Par Achievement Award 2019 for her contributions to parallel processing, the DonaTIC award, category Academia/Researcher in 2019 and the HPDC Achievement Award 2021 for her innovations in parallel task-based programming models, workflow applications and systems, and leadership in the high performance computing research community. In 2023, she has been invited to be a member of the Institut d’Estudis Catalans (Catalan academy).